The PionEar sensor concept is based on Syntiant TinyML board. TinyML board is the ideal platform for building low-power voice, acoustic event detection (AED) and sensor ML applications. Equipped with the ultra-low-power Syntiant® NDP101 Neural Decision Processor, the TinyML board packs native neural network computation for the most demanding applications in the lowest power envelope.
The onboard microphone and BMI160 sensor enable easy configuration for any speech, AED or 6-axis motion-and vibration-related application. Trained models can be easily downloaded on the TinyML board through a micro USB connection without the need for any specialized hardware.
The below picture shows how the TinyML board is interfaced with other external circuitry. The external custom-designed PCB contains RGB LED for logo illumination, DC to DC power supply, and a phototransistor acting as a light sensor. The light sensor provides a signal (AIN) that is sensed by the TinyML board, which controls the brightness of the logo. This prevents the driver from being dazzled at night but enables good visibility in the daylight.
The high-temperature li-Pol battery cell (Zoncell LP602530T / 500mAh) is connected directly to the TinyML board battery connector. In parallel to the battery, a solar charger module AEMLION and solar panel IXOLAR SM101K07TF are connected as well. The whole system draws less than 2mA so the solar panel is able to deliver enough power to cover the power consumption of the system. This is valid in any daylight condition not necessarily in the sunlight. It is still possible to recharge the battery via a micro USB port which is present on TinyML Board.
I plan to reduce average current consumption even more by leveraging a motion sensor (MEMS accelerometer) and an additional MOSFET switch. It will sense if a car is moving – if not, it will cut off the power supply from the system. This feature aims to prevent battery discharge during periods when the car is idle and lacks adequate solar charging, such as nighttime or when parked in a garage. I'm confident that once this feature is in place, the PionEar sensor might operate without the need for any charging.
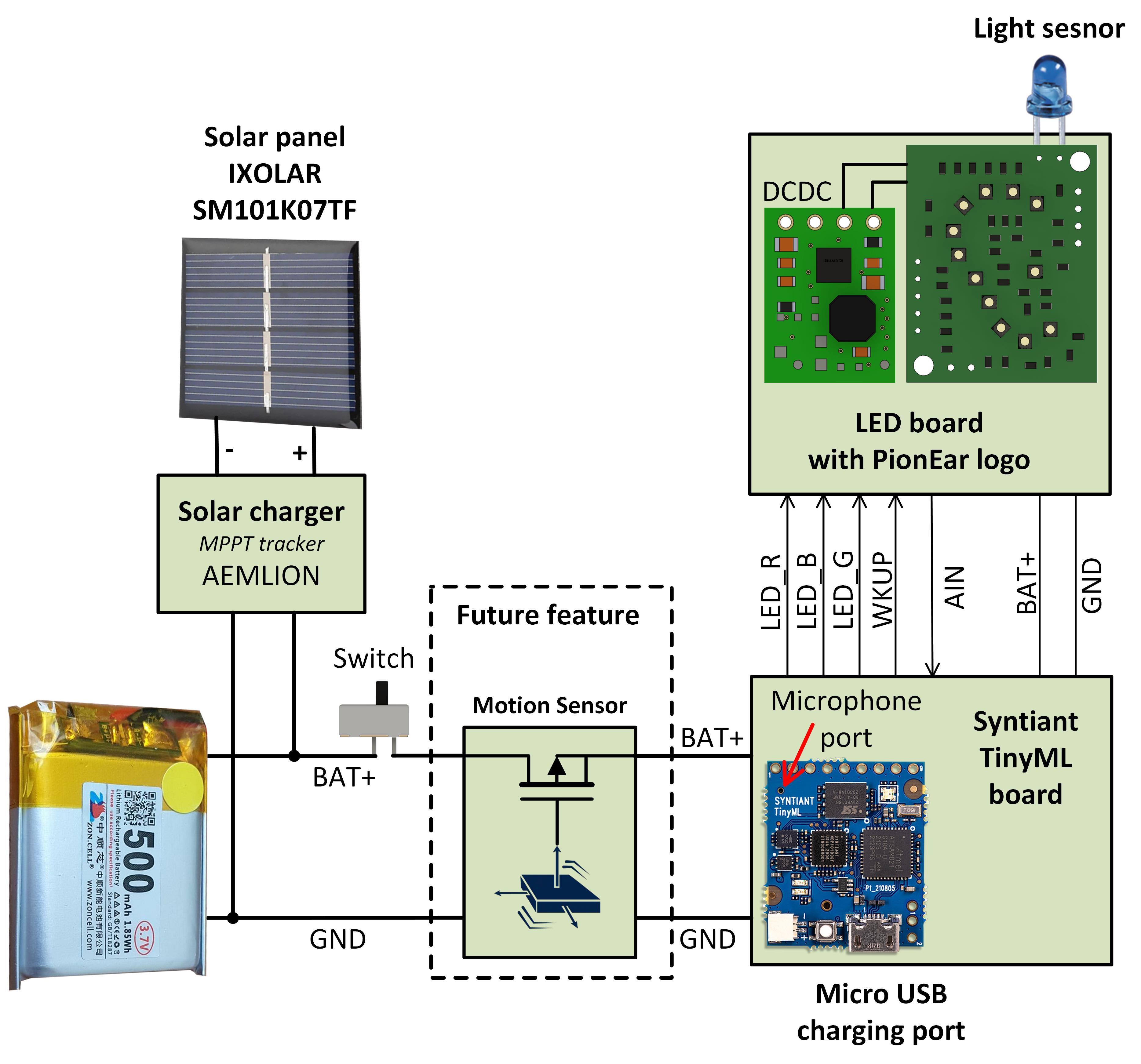
Fig. 1) PionEar sensor HW block diagram
In order to deploy the machine learning model into a TinyML board the Edge Impulse platform can be preferably used. Edge Impulse provides easy to understand interface where you can create and manage your datasets, extract features, train ML models, test and finally deploy into numerous of supported hardware platforms. The TinyML board is fully supported so I have leveraged this advantage.
First, it is necessary to have a suitable dataset of emergency siren sounds and other road noises. One can find many public datasets – I have used sireNNet as a basis for my ML model. I have used other datasets of noises and speech to mix with the original dataset. My dataset including ML model is publicly available on the Edge Impulse platform – you can find it here. I expect, that I will further evolve it as I will continue with the prototype testing.
I have already tested the latest ML model in real driving conditions. It can safely detect ambulance sirens and exhibits a low amount of false positives when exposed to road noises while driving. There are still some weak points for example music when listening to the radio in the car. However, music was not part of the dataset because I expect that hearing-impaired people typically do not listen to the radio in a car 😊
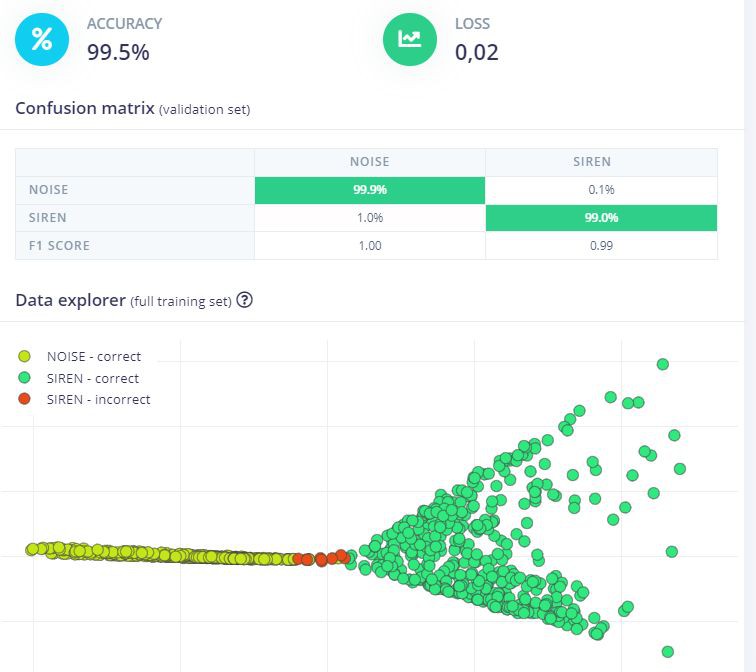
Fig. 2) ML model accuracy for testing dataset
I had been thinking about this sort of project for people in general and more particularly that it would warn if the emergency vehicle was approaching.
I would avoid using a battery powered device as they degrade and can become dangerous in a hot vehicle. May be less of a problem in the UK, but a major issue elsewhere. I don't think there would be a problem with the device being powered via the 12 Volt system plug.